Symposium Report on the Next Decade of Artificial Intelligence
Fostering Opportunities While Regulating Risks | August 5, 2024
Introduction
Generative artificial intelligence (“generative AI”)[1] is rapidly transforming the landscape of artificial intelligence. Unlike AI models that manipulate data for tasks like classification, generative AI creates entirely new content – text, image, audio, and video. There are many ways this technology can help people, from completing routine administrative tasks to assisting with medical developments. While this presents exciting opportunities, there are several risks associated with this technology. It is crucial to timely address these risks before it is too late.
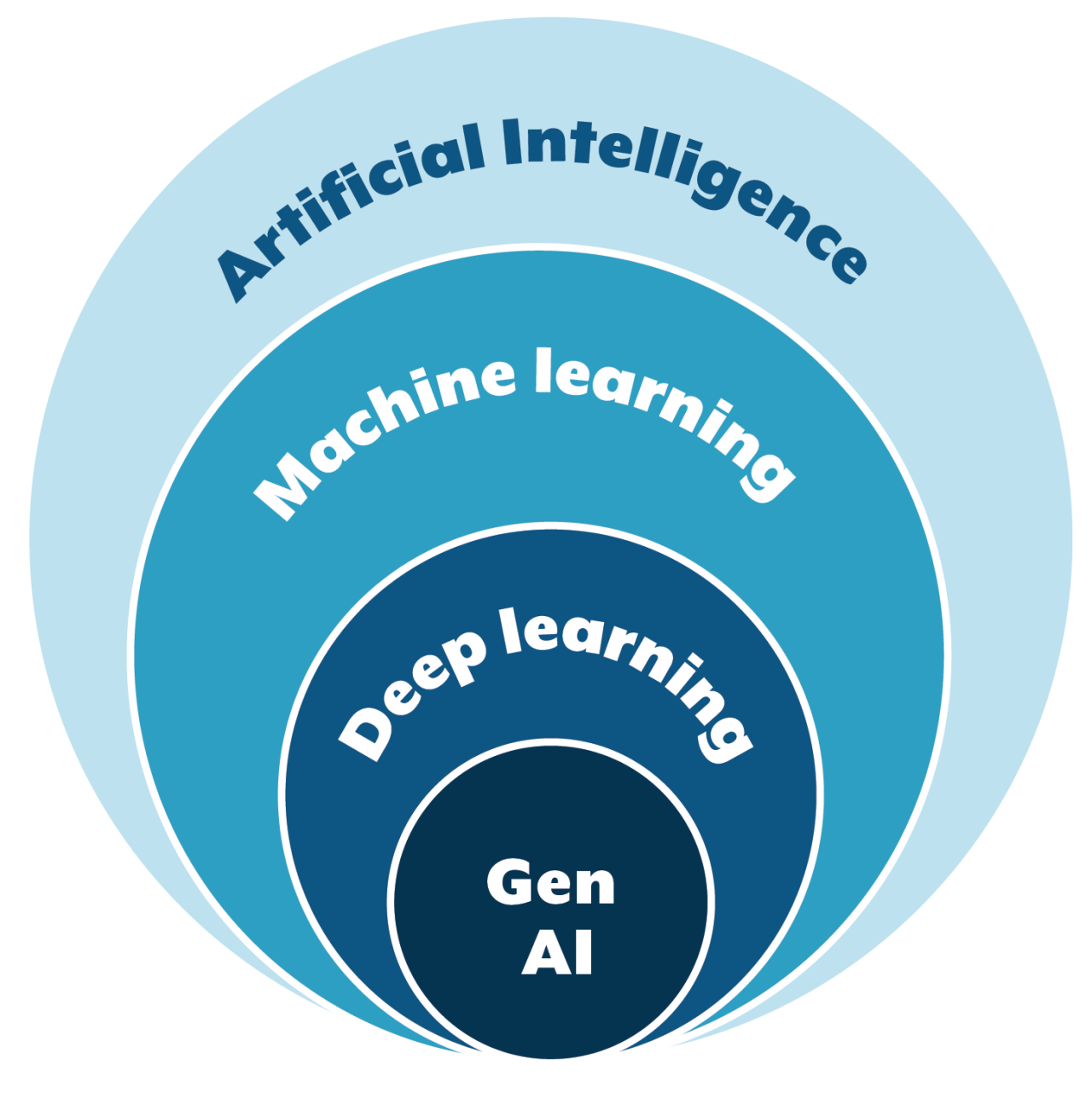
Figure 1: Generative AI is a subset of artificial intelligence that has emerged through developments in machine learning.
On April 12, 2024, the Office of the New York State Attorney General (OAG) hosted The Next Decade of Generative AI: Fostering Opportunities While Regulating Risks. This private symposium brought together leading academics, policymakers, advocates, and industry representatives in panel discussions to address the major opportunities as well as the risks presented by AI technology, especially generative AI. The purpose was to help OAG develop strategies to mitigate those risks while ensuring New York can remain at the forefront of innovation. Although generative AI was a particular focus, speakers also addressed more traditional AI technology, such as automated decision-making technology.[2]
This report outlines the key takeaways we learned from the symposium. It is intended to share insights with other policymakers and government agencies, and to facilitate a public dialogue on developing legal and policy approaches to AI technology.
Opportunities and risks
Over the course of the day, speakers identified several areas where AI technology, including generative AI, may provide meaningful benefits for the public, as well as the major risks that the technology poses.
Healthcare uses
AI technology has the potential to improve healthcare. Participants at the symposium discussed how AI can be used for early disease detection; drug discovery; monitoring trends in public health; administrative tasks that can alleviate physician burnout; and precision medicine, which involves the creation of personalized treatment plans based on information like genetic and clinical profiles.
AI tools have already been used to assist with medical imaging, making scans faster and less expensive. These tools can help clinicians triage by screening medical images to identify potentially urgent issues for priority review by a physician. AI models are now trained to go a step further and help detect disease. A speaker discussed an AI tool that can review mammograms and identify abnormalities that could signal breast cancer risk up to five years prior to developing cancer, allowing for earlier intervention and potentially better outcomes.[3] Speakers agreed that such AI tools should be used to augment clinicians’ work rather than replace it.
On the administrative front, AI is now used to help ease the burden on clinicians, such as by transcribing patient conversations. A physician discussed attempts to use generative AI technology to summarize patient histories to help ensure clinicians see relevant information that might otherwise get lost in extensive notes. This speaker noted that generative AI tools can also create responses to simple patient questions via chat and can provide translation services. As the technology develops, he observed, AI tools could continuously be running in hospital surroundings. For example, recording tools could be used to transcribe patient conversations or monitoring tools could continuously observe vital signs in patients’ rooms. Such tools could potentially be used in patients’ homes, such as video to monitor patient activity.
However, these developments come with risks. Healthcare data is especially sensitive. Patients may not understand what data is being collected or how it is being used by AI tools, especially when such tools are continuously running in their hospital rooms or even homes. In addition to these privacy concerns, there are also serious concerns about unequal access. Minority groups are underrepresented in clinical data used to create personalized treatment plans, and AI transcription services currently do not cover a broad range of languages or accents. To effectively use AI tools in such a sensitive context, speakers noted, there must be a human involved who has ultimate responsibility and who is prepared to make decisions on when to trust AI tools and when to challenge them.
Information and misinformation
AI tools, including chatbots powered by generative AI, can help people easily find information. For example, they are already being used to supplement some phone lines, such as 311 public non-emergency services and corporate customer service. This use of chatbots can free up phone operators to focus on providing specific services and addressing complicated questions. In addition, generative AI tools can automate translation, allowing government and businesses to better communicate with people in their native languages and provide better access to information.
However, as multiple speakers noted, the technology is far from perfect. Generative AI is notoriously prone to arriving at faulty conclusions, or “hallucinations,” and providing false responses. Generative AI chatbots can therefore share incorrect information with people, making them a flawed tool for providing information to the public. These chatbots can also fabricate stories about people, which could cause emotional and reputational harm.
In addition, generative AI can be used by bad actors to intentionally create misinformation materials, such as deepfakes. Laws around defamation and fraud provide some recourse but do not address the full scope of the problem, particularly as deepfakes become increasingly realistic and harder to detect. Speakers noted that the use of generative AI in misinformation would be a major concern over the coming months ahead of the general election, as bad actors may create a deluge of misinformation that cannot be adequately factchecked in time. They cited examples of audio and visual deepfakes that could have serious repercussions if people believed they were true, such as robocalls imitating presidential candidates that encouraged people not to vote in primary elections,[4] images of former President Trump embracing Dr. Fauci,[5] and an image of an explosion at the Pentagon that briefly interrupted markets.[6]
Administrative tasks and automated decision-making
AI tools may be helpful to streamline a host of administrative tasks, particularly for government agencies. For example, a government official outlined opportunities to use generative AI to calculate tax liability, generate public education materials, and write computer code.
One common use case for AI technology is to assist with reviewing applications, which can significantly streamline those processes. For example, by using AI tools to automatically identify people eligible for services or benefits, government agencies can distribute those services and benefits to constituents more quickly and efficiently.
Of course, using AI tools to prescreen applications also comes with risks. Many companies use AI screening tools for hiring, potentially introducing algorithmic bias. One researcher noted that some companies may have started to use AI tools in hiring with the goal of addressing the unfairness and implicit bias inherent in human review. However, speakers cited ample evidence that AI tools often amplify, rather than correct, bias. For example, algorithms trained on data from past hiring can amplify human biases reflected in past hiring decisions and entrench existing norms. The black-box nature of AI algorithms makes it difficult to understand whether and how AI tools work, making it difficult to ensure fairness in decision making. In fact, a speaker argued that it is best to assume that AI tools discriminate by default.
Data concerns
As generative AI models are trained on unprecedentedly vast data sets, the quality, quantity, and fair use of training data raise several concerns. A key issue is copyright, as companies are using copyrighted articles, images, and videos collected from across the internet in their models without compensating the creators for their work. Copyright concerns have received much public attention and are currently being litigated. Another key issue, discussed in the context of healthcare in a previous section, is the underrepresentation of minority groups in training data. As a result, generative AI tools may create outputs that benefit only certain groups.
There are also other data concerns that have not received as much attention, such as the availability of data used to train AI models. Generative AI models need vast amounts of data for training. Consequently, companies that had been scraping the web for years for free have an enormous advantage over newer entrants to the AI market. This is particularly true as platforms and content providers have started to lock up their data and enter into exclusive licensing agreements. This situation raises concerns that the market will become concentrated around just a few players, suppressing competition and further innovation while the technology is still in its infancy.
“Data democratization,” or encouraging the free flow of data, may allow for greater innovation. Of course, any such initiatives should be balanced with privacy concerns, especially concerning sensitive data. As companies seek additional data for training, models are increasingly using their own outputs for training, called “synthetic data.” The use of synthetic data may reinforce issues, particularly with hallucinations, and ultimately cause models to become more error-prone (“model collapse”).
There are also concerns about generative AI tools outputting content that is false, biased, or otherwise problematic because the model was trained on data that was itself flawed. This is often referred to as the “garbage in, garbage out” problem. Because there is little transparency into how AI models operate, one speaker noted concerns with outputs that may have been trained on inaccurate data (e.g., farcical articles), inappropriate data (e.g., protected classes like race or sex), or secret data (e.g., trade secrets). Another speaker warned that inadequate privacy protections on training data may allow generative AI tools to leak personal data or reidentify deidentified data in their outputs.
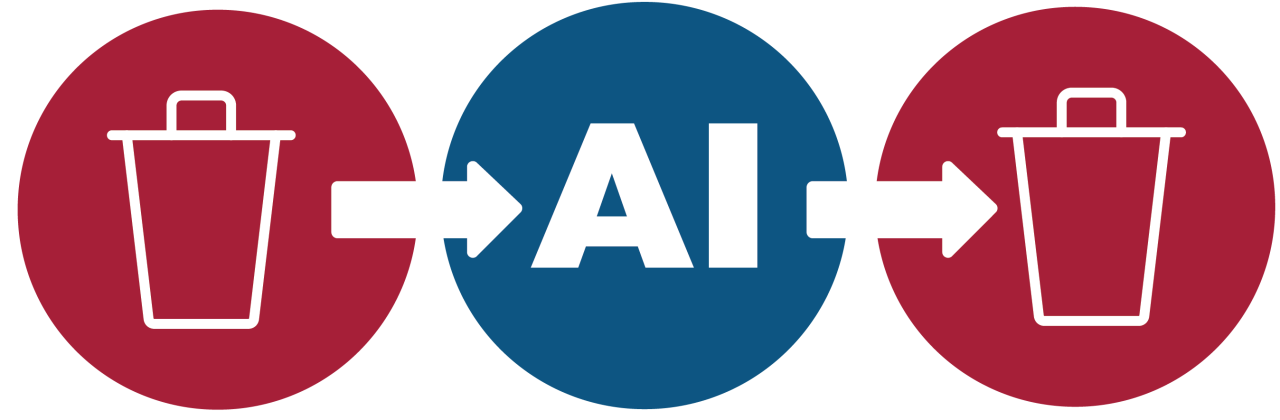
Figure 2: Garbage data in produces garbage data out.
Mitigation strategies
Throughout the day, speakers suggested a host of strategies to effectively utilize generative AI while mitigating the risks associated with the technology.
Public adoption and education
Many people are overly confident that AI tools will solve many problems and yet simultaneously suspicious of those same tools, which deters AI adoption in many spheres. However, AI tools, especially generative AI tools, by their nature require adoption and testing to improve. There is also some education that occurs through adoption. It helps people understand how AI technology works, both in its uses and limits, and helps dispel common myths. Several speakers warned that, for high-risk uses of AI technology, it was important to have a “human in the loop,” meaning having a human actively involved in setting up, testing, and adjusting AI models. In lower-risk scenarios, however, broader adoption of AI tools could help prepare employees to take on such roles.
A former policymaker pointed out that, because generative AI is a general-purpose technology with yet-unknown uses, consumers must understand the technology and evolving applications to ensure they are not vulnerable to misuses, like phishing scams. Speakers also discussed the importance of public engagement and of providing ways for the public to express their views and provide feedback on AI use cases, including for hiring and government use.
Greater public education on generative AI is crucial to mitigate the impact of potential misuses. As discussed previously, many expect generative AI tools to play a major role in spreading misinformation ahead of elections. Speakers emphasized that public education on identifying AI-generated content should be a top priority before a consequential event, such as elections.
Transparency and auditing
Throughout the day, speakers repeatedly called for greater transparency in the use of AI. Most importantly, consumers should know when they are interacting with generative AI tools and when they are encountering AI-generated content. To this end, speakers recommended adding clear disclosures to consumers in a variety of ways: plain-language data use policies that explain what data is being collected and why, how it will be protected, and how it will be used; notice when communicating with a chatbot, which is already mandated by law in some states; and conspicuous labels or watermarks on AI-generated content. While some argue that watermarks may be easy to manipulate by sophisticated bad actors, one speaker noted that it would still be beneficial in most circumstances and at least would slow down bad actors intentionally trying to deceive people. Therefore, multiple speakers called for a robust watermarking framework.
Currently, there is little transparency into how AI models are audited. By nature, AI algorithms are not transparent; therefore, auditing of traditional AI tools often focuses on assessing the outputs created to identify issues, such as bias. However, speakers noted that auditing is largely done ad hoc, and companies and researchers may not explain how they conduct audits. To address this issue, speakers called for clear standards and procedures around auditing models.
There is some precedent for such standards, such as New York City Local Law 144[7] and its implementing rules, which outline minimum requirements for a bias audit that must be done when using automated decision-making technology (ADMT) for hiring. Similarly, financial institutions have developed robust fair lending compliance programs that assess and manage bias in algorithmic underwriting frameworks. Additionally, one speaker noted that auditing should be context specific. For example, when auditing a model for election misinformation, an election commissioner should provide expert guidance on what information is or is not correct. A second speaker suggested creating professional certifications for algorithm auditors to increase trust in the process. Finally, a third speaker called for greater access for outside researchers to audit AI models.
Consumer rights
Consumers should feel empowered when it comes to AI tools. A former government official cited the White House’s Blueprint for an AI Bill of Rights[8] as a good starting place for efforts to establish clear consumer rights. The blueprint outlines five areas where consumers should be afforded protections from AI tools, including safety, discrimination, and data privacy. In addition, the blueprint addresses the importance of transparency and giving users the right to opt out of the use of ADMT in favor of a human decisionmaker.

Figure 3: The White House’s Blueprint for an AI Bill of Rights identifies five key principles.[9]
California is currently adopting similar principles in its rulemaking on ADMT. A California state official discussed the rulemaking process in depth, including the importance of providing consumers the ability to opt out of the use of ADMT for significant decisions, or at least the ability to appeal such decisions to a qualified human decisionmaker.
Regulation and oversight
While technology changes rapidly, it may seem that laws are slow to follow, but speakers discussed many existing laws that apply to the use of AI technology. Laws around discrimination, civil liberties, privacy, data security, defamation, fraud, deception, and competition can be used to rein in some of the potential harms associated with AI technology. Speakers also noted New York’s efforts to regulate algorithmic harms, such as New York City Local Law 144 discussed previously, and the SAFE for Kids Act,[10] which regulates social media platforms’ ability to present addictive algorithmic feeds to children.
Speakers generally agreed government must have greater oversight over AI technology, even without a perfect understanding of the technology. Government can regulate agency use of AI tools and use procurement as a lever for regulation, such as through the White House Executive Order on the Safe, Secure, and Trustworthy Development and Use of Artificial Intelligence[11] and California’s executive order on the state’s use of generative AI.[12]
However, speakers had differing views on how to approach broader regulation of AI technology. Some favored the passage of a comprehensive law, such as the European Union’s Artificial Intelligence Act (EU AI Act), [13] which creates a broad framework of regulation based on risk and establishes a centralized agency to oversee AI technology. Other speakers argued such a model is not appropriate in the U.S., and instead advocated for regulation and oversight to be divided by sector and handled within separate agencies. This would mean, for example, that the Department of Health and Human Services could be the primary regulator of AI technology issues associated with healthcare.
The latter group noted that distributed authority would allow agencies to be nimbler in adapting regulations to changing technology and would allow for more competition and innovation. Proponents of a comprehensive regulatory regime countered that sector-specific regulation along with state and local laws can complement a broad framework. Notably, the leader of an advocacy organization warned not to believe that we must choose between prudent adoption or innovation, as government’s responsibility is to maximize both.
Developments and areas for further inquiry
Since the symposium, there have been significant developments in regulating AI technology. New York enacted the SAFE for Kids Act, discussed previously, a major steppingstone to protecting children from algorithmic harms online.
Other jurisdictions have also been active in recent months. In May, Colorado enacted the Colorado Artificial Intelligence Act, [14] which, much like the EU AI Act, imposes obligations on the use of AI tools based on the risk of harm to consumers. In that same month, the U.S. Senate issued a roadmap for AI policy, which calls for $32 billion in funding for AI innovation and legislation to supplement existing laws that apply to AI technology.[15] In July, the Federal Trade Commission, U.S. Department of Justice, and EU and UK competition authorities issued a joint statement outlining principles to protect competition in the AI ecosystem.[16]
However, as New York prepares to tackle the risks of AI technology, and particularly generative AI, there are issues to further study and understand. For example, multiple speakers called for algorithmic auditing standards, but there is no consensus on the appropriate standard nor how auditing approaches used for traditional AI tools may be adopted for auditing generative AI models. In a similar vein, there is no consensus on how to develop a robust watermarking framework for AI-generated content. Since these types of issues require technical expertise, there remain questions on how to ensure the appropriate people are involved in developing such standards and frameworks.
In addition, as noted previously, there is disagreement on the appropriate framework for regulating AI technology, including the proper level of centralization. The OAG is actively monitoring the effectiveness of different regulatory frameworks, like the EU AI Act, to inform future legislative and regulatory proposals.
The OAG will continue to listen and learn about this developing technology and the appropriate ways to encourage innovation while protecting New Yorkers.
[1] Generative AI is a subset of artificial intelligence (AI) that generates content like text, images, audio, and video based on a prompt. Generative AI models are trained on vast data sets and have developed through advances in deep learning, a subset of machine learning. A resource to learn more about the basics of artificial intelligence and machine learning is Abail, I.E., et al. (2023). Technology Primer for Policymakers: Artificial Intelligence & Machine Learning. Belfer Center for Science and International Affairs.
[2] References to “AI tools,” “AI models,” and “AI technology” in this report may refer to traditional machine learning models or a combination of traditional machine learning and generative AI technology.
[3] Yala, A., et al. (2021, Jan. 27). Toward Robust Mammography-Based Models for Breast Cancer Risk. Science Translational Medicine, 13(578).
[4] Astor, M. (2024, May 23). Political Consultant Who Orchestrated Fake Biden Robocalls Is Indicted. The New York Times.
[5] Nehamas, N. (2023, June 8). DeSantis Campaign Uses Apparently Fake Images to Attack Trump on Twitter. The New York Times.
[6] Marcelo, P. (2023, May 23). Fact Focus: Fake Image of Pentagon Explosion Briefly Sends Jitters Through Stock Market. Associated Press.
[7] New York City Administrative Code section 20-870 et seq.
[8] Office of Science and Technology Policy, Executive Office of the President. (2022, October). Blueprint for an AI Bill of Rights.
[9] See note 8.
[10] New York General Business Law section 1500 et seq. The SAFE for Kids Act was being considered in the legislature at the time of the symposium and was subsequently enacted on June 20, 2024.
[11] Executive Order No. 14110, 88 Fed. Reg. 75191 (2023, October 30).
[12] California Executive Order N-12-23 (2023, September 6).
[13] Regulation (EU) 2024/1689 of the European Parliament and of the Council of 13 June 2024 laying down harmonised rules on artificial intelligence and amending Regulations (EC) No 300/2008, (EU) No 167/2013, (EU) No 168/2013, (EU) 2018/858, (EU) 2018/1139 and (EU) 2019/2144 and Directives 2014/90/EU, (EU) 2016/797 and (EU) 2020/1828 (Artificial Intelligence Act).
[14] Colorado Revised Statutes section 6-1-1706 et seq.
[15] Bipartisan Senate AI Working Group. (2024, May). Driving U.S. Innovation in Artificial Intelligence: A Roadmap for Artificial Intelligence Policy in the U.S. Senate.
[16] Federal Trade Commission. (2024, July 23). Joint Statement on Competition in Generative AI Foundation Models and AI Products.